Descriptive Statistics Interview Questions
- Home
- »
- Descriptive Statistics Interview Questions
- What is meant by qualitative data?
- Define quantitative data.
- What is the difference between discrete and continuous data?
- Give an example of qualitative data.
- Provide an example of quantitative data.
- What are the two subtypes of qualitative data?
- Define nominal data.
- Give an example of nominal data.
- What is ordinal data?
- Provide an example of ordinal data.
- Define discrete data.
- Give an example of discrete data.
- What is continuous data?
- Provide an example of continuous data.
- What is the difference between interval and ratio data?
- What is central tendency?
- What are the common measures of central tendency?
- Define the mean.
- Define the median.
- When is the median the most appropriate measure of central tendency?
- Define the mode.
- When is the mode the most appropriate measure of central tendency?
- What is the relationship between the mean, median, and mode in a symmetric distribution?
- How does the mean change if an extreme outlier is added to a dataset?
- What does a large range or interquartile range suggest about the data?
- Can the median be affected by extreme outliers?
- How do you calculate the weighted mean?
- When is the weighted mean used?
- How does the mean change if values in a dataset are multiplied or divided by a constant?
- What is a measure of spread in statistics?
- What are the common measures of spread?
- Define the range.
- Define the variance.
- Define the standard deviation.
- Define the standard deviation.
- When is the standard deviation more appropriate to use than the range?
- Define the interquartile range (IQR).
- What does a small standard deviation indicate?
- What does a correlation coefficient of -1 indicate?
- What does a correlation coefficient of 0 indicate?
- What does a correlation coefficient of 1 indicate?
- What is the coefficient of determination (R-squared)?
- What is the difference between correlation and causation?
- What is the sample space in set theory?
- What is a subset in set theory?
- What is the complement of a set?
- What is the union of two sets?
- What is the intersection of two sets?
- What is the difference between two sets?
- What is the empty set or null set?
- What is the cardinality of a set?
- What is the principle of inclusion-exclusion in set theory?
- What is the concept of mutually exclusive sets?
- What is Naive Bayes?
- What is the underlying principle of Naive Bayes?
- How does Naive Bayes handle continuous and categorical features?
- What is the main advantage of Naive Bayes?
- Can Naive Bayes handle missing values in the dataset?
- How does Naive Bayes handle zero probabilities?
- What are the different types of Naive Bayes classifiers?
- What are the different types of Naive Bayes classifiers?
- What is Laplace smoothing in Naive Bayes?
- WCan Naive Bayes handle continuous target variables?
- What is a decision tree?
- What are the advantages of using decision trees?
- How does a decision tree determine which feature to split on?
- What is the formula for updating the trend component in Holt's exponential smoothing?
- What is pruning in decision trees?
- Can decision trees handle missing values in the dataset?
- How does a decision tree handle continuous features?
- What is information gain in decision trees?
- What is Gini impurity in decision trees?
- How does a decision tree handle categorical features?
- What is imbalanced machine learning?
- What are the challenges of working with imbalanced datasets?
- What are some common techniques to address imbalanced datasets?
- What is oversampling and how does it help with imbalanced datasets?
- What is undersampling and how does it help with imbalanced datasets?
- What is the difference between oversampling and undersampling?
- What is the concept of SMOTE (Synthetic Minority Over-sampling Technique)?
- What is the impact of imbalanced datasets on evaluation metrics like accuracy?
- What is the concept of cost-sensitive learning in imbalanced machine learning?
- What is the impact of data quality on handling imbalanced datasets?
- What is imbalanced machine learning?
- What are the challenges of working with imbalanced datasets?
- What are some common techniques to address imbalanced datasets?
- What is oversampling and how does it help with imbalanced datasets?
- What is undersampling and how does it help with imbalanced datasets?
- What is the difference between oversampling and undersampling?
- What is the concept of SMOTE (Synthetic Minority Over-sampling Technique)?
- What is the impact of imbalanced datasets on evaluation metrics like accuracy?
- What is the concept of cost-sensitive learning in imbalanced machine learning?
- What is the impact of data quality on handling imbalanced datasets?
- What is imbalanced machine learning?
- What are the challenges of working with imbalanced datasets?
- What are some common techniques to address imbalanced datasets?
- What is oversampling and how does it help with imbalanced datasets?
- What is undersampling and how does it help with imbalanced datasets?
- What is the difference between oversampling and undersampling?
- What is the concept of SMOTE (Synthetic Minority Over-sampling Technique)?
- What is the impact of imbalanced datasets on evaluation metrics like accuracy?
- What is the concept of cost-sensitive learning in imbalanced machine learning?
- What is the impact of data quality on handling imbalanced datasets?
- What is imbalanced machine learning?
- What are the challenges of working with imbalanced datasets?
- What are some common techniques to address imbalanced datasets?
- What is oversampling and how does it help with imbalanced datasets?
- What is undersampling and how does it help with imbalanced datasets?
- What is the difference between oversampling and undersampling?
- What is the concept of SMOTE (Synthetic Minority Over-sampling Technique)?
- What is the impact of imbalanced datasets on evaluation metrics like accuracy?
- What is the concept of cost-sensitive learning in imbalanced machine learning?
- What is the impact of data quality on handling imbalanced datasets?
What is meant by qualitative data?
Answer: Qualitative data is non-numerical data that describes qualities or characteristics. It is typically categorical or ordinal in nature.
Define quantitative data.
Answer: Quantitative data is numerical data that can be measured or counted. It is typically continuous or discrete in nature.
What is the difference between discrete and continuous data?
Answer: Discrete data can only take on specific values, typically whole numbers, while continuous data can take on any value within a given range.
Give an example of qualitative data.
Answer: Examples of qualitative data include gender, marital status, eye color, or customer satisfaction rating (e.g., “good,” “fair,” “excellent”).
Provide an example of quantitative data.
Answer: Examples of quantitative data include height, weight, age, temperature, or income.
What are the two subtypes of qualitative data?
Answer: The two subtypes of qualitative data are nominal and ordinal data.
Define nominal data.
Answer: Nominal data is a type of qualitative data that consists of categories with no inherent order or ranking.
Give an example of nominal data.
Answer: Examples of nominal data include favorite color (e.g., red, blue, green), car brands (e.g., Ford, Toyota, Honda), or blood types (e.g., A, B, AB, O).
What is ordinal data?
Answer: Ordinal data is a type of qualitative data that has categories with a natural order or ranking.
Provide an example of ordinal data.
Answer: Examples of ordinal data include rating scales (e.g., “poor,” “fair,” “good,” “excellent”), educational levels (e.g., high school, bachelor’s, master’s, Ph.D.), or military ranks (e.g., private, sergeant, lieutenant).
Define discrete data.
Answer: Discrete data consists of separate, distinct values that can be counted and are typically whole numbers.
Give an example of discrete data.
Answer: Examples of discrete data include the number of children in a family, the number of cars in a parking lot, or the number of students in a classroom.
What is continuous data?
Answer: Continuous data is data that can take on any value within a certain range and can be measured on a continuous scale.
Provide an example of continuous data.
Answer: Examples of continuous data include height, weight, temperature, time, or the amount of rainfall.
What is the difference between interval and ratio data?
Answer: Interval data has a meaningful order and equal intervals between values, but it doesn’t have a true zero point. Ratio data, on the other hand, has a true zero point.
Give an example of interval data.
Answer: Examples of interval data include temperature measured in Celsius or Fahrenheit, or years (e.g., 1990, 2000, 2010).
What is ratio data?
Answer: Ratio data is a type of quantitative data that has a true zero point and meaningful ratios between values.
Provide an example of ratio data.
Answer: Examples of ratio data include height, weight, time, income, or distance.
What are the levels of measurement in statistics?
Answer: The levels of measurement are nominal, ordinal, interval, and ratio.
What is the purpose of regularization in linear regression?
Answer: Regularization is used to prevent overfitting by adding a penalty term to the error function, which helps to shrink the coefficients towards zero.
Central Tendency
What is central tendency?
Answer: Central tendency refers to the measure that represents the typical or central value of a dataset.
What are the common measures of central tendency?
Answer: The common measures of central tendency are the mean, median, and mode.
Define the mean.
Answer: The mean is the sum of all values in a dataset divided by the number of values. It represents the average value.
Define the median.
Answer: The median is the middle value in a dataset when the values are arranged in ascending or descending order. It divides the dataset into two equal halves.
When is the median the most appropriate measure of central tendency?
Answer: The median is most appropriate when the dataset contains outliers or is skewed.
Define the mode.
Answer: The mode is the value or values that occur most frequently in a dataset.
When is the mode the most appropriate measure of central tendency?
Answer: The mode is most appropriate for categorical or discrete data, or when identifying the most common value is of interest.
What is the relationship between the mean, median, and mode in a symmetric distribution?
Answer: In a symmetric distribution, the mean, median, and mode are approximately equal.
How does the mean change if an extreme outlier is added to a dataset?
Answer: The mean is sensitive to outliers, so adding an extreme outlier can significantly change the value of the mean.
Can a dataset have multiple modes?
Answer: Yes, a dataset can have one mode (unimodal), two modes (bimodal), or more than two modes (multimodal) if there are multiple values with the same highest frequency.
Can the median be affected by extreme outliers?
Answer: No, the median is not affected by extreme outliers as it only considers the middle value(s) in the dataset.
How do you calculate the weighted mean?
Answer: The weighted mean is calculated by multiplying each value by its corresponding weight, summing the products, and dividing by the sum of the weights.
When is the weighted mean used?
Answer: The weighted mean is used when different values in the dataset have different importance or significance.
How does the mean change if values in a dataset are multiplied or divided by a constant?
Answer: Multiplying or dividing all values in a dataset by a constant will result in the mean being multiplied or divided by the same constant.
Measures of Spread and Dependence
What is a measure of spread in statistics?
Answer: A measure of spread, also known as a measure of dispersion, quantifies the extent to which data values are spread out or clustered together.
What are the common measures of spread?
Answer: The common measures of spread are the range, variance, standard deviation, and interquartile range (IQR).
Define the range.
Answer: The range is the difference between the largest and smallest values in a dataset.
Define the variance.
Answer: The variance measures the average squared deviation of each data point from the mean. It indicates how much the data values deviate from the mean.
Define the standard deviation.
Answer: The standard deviation is the square root of the variance. It represents the average amount of variation or dispersion in a dataset.
When is the standard deviation more appropriate to use than the range?
Answer: The standard deviation is more appropriate when the distribution of data is approximately symmetrical and follows a bell-shaped curve.
Define the interquartile range (IQR).
Answer: The interquartile range is the difference between the third quartile (Q3) and the first quartile (Q1) in a dataset. It represents the spread of the middle 50% of the data.
What does a small standard deviation indicate?
Answer: A small standard deviation indicates that the data values are closely clustered around the mean, suggesting less variability.
What does a large range or interquartile range suggest about the data?
Answer: A large range or interquartile range suggests that the data values are spread out or have a greater dispersion.
What is correlation?
Answer: Correlation measures the strength and direction of the linear relationship between two variables.
What does a correlation coefficient of -1 indicate?
Answer: A correlation coefficient of -1 indicates a perfect negative linear relationship between two variables.
What does a correlation coefficient of 0 indicate?
Answer: A correlation coefficient of 0 indicates no linear relationship between two variables.
What does a correlation coefficient of 1 indicate?
Answer: A correlation coefficient of 1 indicates a perfect positive linear relationship between two variables.
What is the coefficient of determination (R-squared)?
Answer: The coefficient of determination represents the proportion of the variance in the dependent variable explained by the independent variable(s).
What is the difference between correlation and causation?
Answer: Correlation measures the statistical relationship between variables, while causation establishes a cause-and-effect relationship between variables. Correlation does not imply causation.
Fundamentals of Probability
Basic Probability
What is probability?
Answer: Probability is a measure of the likelihood of an event occurring. It quantifies the uncertainty associated with an outcome.
What is the range of probability values?
Answer: Probability values range from 0 to 1, where 0 indicates an impossible event and 1 indicates a certain event.
What is the difference between theoretical probability and experimental probability?
Answer: Theoretical probability is based on mathematical calculations and assumptions, while experimental probability is determined through actual observations or experiments.
What is the probability of an event that is certain to happen?
Answer: The probability of a certain event is 1.
What is the probability of an event that is impossible to happen?
Answer: The probability of an impossible event is 0.
What is the complement of an event?
Answer: The complement of an event is the probability of that event not occurring. It is calculated as 1 minus the probability of the event.
What is the addition rule of probability?
Answer: The addition rule states that the probability of the union of two mutually exclusive events is equal to the sum of their individual probabilities.
What is the multiplication rule of probability?
Answer: The multiplication rule states that the probability of the intersection of two independent events is equal to the product of their individual probabilities.
What is conditional probability?
Answer: Conditional probability is the probability of an event occurring given that another event has already occurred. It is denoted as P(A|B), where A and B are events.
What is the difference between independent and dependent events?
Answer: Independent events are events that do not affect each other’s probability, while dependent events are events that do affect each other’s probability.
What is the concept of sample space?
Answer: The sample space is the set of all possible outcomes of a random experiment.
How do you calculate the probability of an event in a discrete uniform distribution?
Answer: In a discrete uniform distribution, where all outcomes are equally likely, the probability of an event is calculated by dividing the number of favorable outcomes by the total number of outcomes.
What is the concept of mutually exclusive events?
Answer: Mutually exclusive events are events that cannot occur simultaneously. If one event happens, the other event cannot occur.
What is the concept of independent events?
Answer: Independent events are events that are not influenced by each other. The occurrence or non-occurrence of one event does not affect the probability of the other event.
How do you calculate the probability of the union of two events?
Answer: The probability of the union of two events A and B is calculated by adding the probabilities of A and B and subtracting the probability of their intersection (A ∩ B).
Set Theory
What is the sample space in set theory?
Answer: The sample space is the set of all possible outcomes in an experiment or event.
What is a subset in set theory?
Answer: A subset is a set that contains only elements that are also found in another set.
What is the complement of a set?
Answer: The complement of a set is the set of all elements that are not in the given set, denoted as A’.
What is the union of two sets?
Answer: The union of two sets is the set that contains all elements that are in either of the two sets, denoted as A ∪ B.
What is the intersection of two sets?
Answer: The intersection of two sets is the set that contains all elements that are common to both sets, denoted as A ∩ B.
What is the difference between two sets?
Answer: The difference between two sets is the set that contains all elements that are in the first set but not in the second set, denoted as A – B.
What is the empty set or null set?
Answer: The empty set or null set is a set that contains no elements, denoted as ∅.
What is the cardinality of a set?
Answer: The cardinality of a set is the number of elements it contains. It is denoted as |A|, where A is the set.
What is the principle of inclusion-exclusion in set theory?
Answer: The principle of inclusion-exclusion is a formula used to calculate the size of the union of multiple sets.
What is the concept of mutually exclusive sets?
Answer: Mutually exclusive sets are sets that have no common elements. If one set has an element, the other set cannot have the same element.
What is the concept of disjoint sets?
Answer: Disjoint sets are sets that have no common elements. They are also mutually exclusive sets.
What is the concept of the power set?
Answer: The power set of a set is the set of all possible subsets of that set, including the empty set and the set itself.
What is the concept of the Cartesian product?
Answer: The Cartesian product of two sets A and B is the set of all ordered pairs where the first element comes from set A and the second element comes from set B.
What is the concept of a proper subset?
Answer: A proper subset is a subset that contains some, but not all, elements of another set.
What is the concept of a universal set?
Answer: The universal set is the set that contains all possible elements or outcomes of a particular problem or scenario. It is typically denoted as Ω or U.
Conditional Probability
What is conditional probability?
Answer: Conditional probability is the probability of an event occurring given that another event has already occurred.
How is conditional probability calculated?
Answer: Conditional probability is calculated by dividing the probability of the intersection of two events by the probability of the condition event.
What is the formula for conditional probability?
Answer: The formula for conditional probability is P(A | B) = P(A ∩ B) / P(B), where P(A | B) represents the conditional probability of event A given event B.
What is the concept of independence in conditional probability?
Answer: Two events A and B are independent if the occurrence of one event does not affect the probability of the other event.
What is the concept of dependence in conditional probability?
Answer: Mutually exclusive events are events that cannot occur at the same time. If one event happens, the other event cannot happen.
What is the concept of mutually exclusive events in conditional probability?
Answer: Mutually inclusive events are events that can occur at the same time. The occurrence of one event does not exclude the possibility of the other event happening.
How is the probability of independent events calculated?
Answer: The probability of independent events is calculated by multiplying the probabilities of each individual event.
How is the probability of dependent events calculated?
Answer: The probability of dependent events is calculated using conditional probability. The probability of the second event is calculated based on the outcome of the first event.
What is the concept of a conditional probability table?
Answer: A conditional probability table is a table that shows the probabilities of different events given certain conditions.
What is the concept of a joint probability?
Answer: Joint probability is the probability of two events occurring together, denoted as P(A ∩ B).
What is the concept of a marginal probability?
Answer: Marginal probability is the probability of a single event occurring without considering any other events.
What is the concept of a prior probability?
Answer: Prior probability is the probability of an event occurring before any additional information is taken into account.
What is the concept of a posterior probability?
Answer: Posterior probability is the updated probability of an event occurring after additional information or evidence is considered.
How is conditional probability used in Bayes' Theorem?
Answer: Bayes’ Theorem is a formula used to calculate the probability of an event given prior knowledge or evidence. It utilizes conditional probability to update the probability based on new information.
Bayes Theorem
What is Bayes' Theorem?
Answer: Bayes’ Theorem is a mathematical formula used to calculate the probability of an event based on prior knowledge or evidence.
What is the formula for Bayes' Theorem?
Answer: The formula for Bayes’ Theorem is P(A|B) = (P(B|A) * P(A)) / P(B), where P(A|B) is the probability of event A given event B, P(B|A) is the probability of event B given event A, P(A) is the prior probability of event A, and P(B) is the prior probability of event B.
How does Naive Bayes handle continuous and categorical features?
Answer: Naive Bayes can handle continuous features using probability density functions such as Gaussian Naive Bayes. For categorical features, it calculates the probabilities directly from the observed frequencies.
What is the importance of Bayes' Theorem in statistics and probability?
Answer: Bayes’ Theorem allows us to update the probability of an event based on new evidence or information, making it a fundamental tool in statistical inference and decision-making.
How does Bayes' Theorem relate to conditional probability?
Answer: Bayes’ Theorem uses conditional probability to calculate the probability of an event given prior knowledge or evidence.
What is the concept of prior probability in Bayes' Theorem?
Answer: Prior probability refers to the initial probability of an event before any additional information or evidence is considered.
What is the concept of posterior probability in Bayes' Theorem?
Answer: Posterior probability is the updated probability of an event after incorporating new evidence or information.
How is Bayes' Theorem applied in medical diagnosis?
Answer: Bayes’ Theorem is used in medical diagnosis to calculate the probability of a particular disease given the observed symptoms and medical test results.
How can Bayes' Theorem be used in spam filtering?
Answer: Bayes’ Theorem can be used in spam filtering to calculate the probability that an incoming email is spam based on the presence of certain keywords or patterns.
What is the relationship between prior probability and posterior probability in Bayes' Theorem?
Answer: The prior probability is updated using Bayes’ Theorem to obtain the posterior probability, which reflects the revised probability after considering new evidence.
What is the role of Bayes' Theorem in machine learning algorithms?
Answer: Bayes’ Theorem is used in various machine learning algorithms, such as Naive Bayes classifiers, to estimate the probability of different outcomes based on observed data.
What are some assumptions made when applying Bayes' Theorem?
Answer: Bayes’ Theorem assumes that the events being considered are independent, and that the prior probabilities are known or can be estimated accurately.
How does Bayes' Theorem handle rare events?
Answer: Bayes’ Theorem can effectively update the probabilities of rare events based on new evidence, allowing for more accurate predictions or estimations.
Can Bayes' Theorem be used with continuous probability distributions?
Answer: Yes, Bayes’ Theorem can be used with continuous probability distributions by integrating over the appropriate ranges of values.
What is the relationship between Bayes' Theorem and the law of total probability?
Answer: Bayes’ Theorem is derived from the law of total probability, which states that the probability of an event can be calculated by considering all possible outcomes and their probabilities.
How does Bayes' Theorem relate to the concept of updating beliefs?
Answer: Bayes’ Theorem provides a framework for updating prior beliefs or probabilities based on new evidence, allowing for a more accurate representation of the true probability of an event.
Permutations and Combinations
What is the difference between permutations and combinations?
Answer: Permutations refer to the arrangement of objects in a particular order, while combinations refer to the selection of objects without considering the order.
What is a permutation?
Answer: A permutation is an arrangement of objects where the order matters.
What is a combination?
Answer: A combination is a selection of objects where the order does not matter.
How many permutations can be formed from a set of n objects taken r at a time?
Answer: The number of permutations is given by nPr = n! / (n – r)!, where n is the total number of objects and r is the number of objects taken at a time.
How many combinations can be formed from a set of n objects taken r at a time?
Answer: The number of combinations is given by nCr = n! / (r! * (n – r)!), where n is the total number of objects and r is the number of objects taken at a time.
What is the factorial function?
Answer: The factorial of a non-negative integer n, denoted by n!, is the product of all positive integers less than or equal to n.
What is the formula for the number of permutations of a set with repetitions?
Answer: The number of permutations of a set with repetitions is given by n1! * n2! * … * nk!, where n1, n2, …, nk are the frequencies of the distinct objects.
How can permutations and combinations be used in probability calculations?
Answer: Permutations and combinations are used to calculate the number of possible outcomes in a probability space, helping to determine the likelihood of specific events.
Can you have repetitions in combinations?
Answer: No, combinations do not involve repetitions. Each object can be selected only once.
Can you have repetitions in permutations?
Answer: Yes, permutations can involve repetitions. Objects can be arranged in a particular order, allowing for repeated elements.
How is the concept of permutations and combinations applied in real-life situations?
Answer: Permutations and combinations are used in various fields, such as probability theory, statistics, cryptography, and combinatorial optimization.
What is the principle of inclusion-exclusion?
Answer: The principle of inclusion-exclusion is a counting technique used to calculate the number of elements in the union or intersection of multiple sets.
How do permutations and combinations relate to Pascal's triangle?
Answer: Pascal’s triangle is a triangular array of numbers where each number represents a combination coefficient. The coefficients in Pascal’s triangle can be used to calculate combinations.
How do permutations and combinations relate to the binomial theorem?
Answer: The binomial theorem provides a way to expand binomial expressions raised to a positive integer power and involves coefficients that correspond to combinations.
What is the concept of sampling with replacement and sampling without replacement in permutations and combinations?
Answer: Sampling with replacement allows for the same object to be selected multiple times, while sampling without replacement restricts each object to be selected only once.
Inferential Statistics
What is inferential statistics?
Answer: Inferential statistics is the branch of statistics that involves making conclusions or predictions about a population based on a sample of data.
What is the difference between descriptive statistics and inferential statistics?
Answer: Descriptive statistics summarizes and describes the characteristics of a sample or population, while inferential statistics makes inferences and draws conclusions about a population based on sample data.
What is a population in inferential statistics?
Answer: In inferential statistics, a population refers to the entire group of individuals or items of interest that we want to study.
What is a sample in inferential statistics?
Answer: In inferential statistics, a sample refers to a subset of individuals or items from a population that is selected to represent the whole population.
What is sampling error?
Answer: Sampling error refers to the discrepancy between the characteristics of a sample and the characteristics of the population it represents. It occurs due to random variation in the sampling process.
What is a hypothesis in inferential statistics?
Answer: A hypothesis is a statement or assumption about a population parameter that is being tested using sample data.
What is a null hypothesis?
Answer: A null hypothesis is a hypothesis that assumes there is no significant difference or relationship between variables in the population.
What is an alternative hypothesis?
Answer: An alternative hypothesis is a hypothesis that contradicts the null hypothesis and suggests that there is a significant difference or relationship between variables in the population.
What is a type I error?
Answer: A type I error occurs when the null hypothesis is rejected, but in reality, it is true. It is also known as a false positive.
What is a type II error?
Answer: A type II error occurs when the null hypothesis is accepted, but in reality, it is false. It is also known as a false negative.
What is a p-value?
Answer: The p-value is the probability of obtaining a test statistic as extreme as, or more extreme than, the observed value, assuming the null hypothesis is true. It is used to assess the strength of evidence against the null hypothesis.
What is a confidence interval?
Answer: A confidence interval is a range of values calculated from sample data that is likely to contain the true population parameter with a certain level of confidence.
What is the significance level?
Answer: The significance level, often denoted as α (alpha), is the threshold below which the null hypothesis is rejected. It determines the probability of committing a type I error.
What is the central limit theorem?
Answer: The central limit theorem states that as the sample size increases, the sampling distribution of the sample mean approaches a normal distribution, regardless of the shape of the population distribution.
What is a point estimate?
Answer: A point estimate is a single value that estimates an unknown population parameter based on sample data.
What is the margin of error?
Answer: The margin of error is the maximum likely difference between the point estimate and the true value of the population parameter.
What is a one-sample t-test?
Answer: A one-sample t-test is a statistical test used to determine whether the mean of a sample is significantly different from a known or hypothesized population mean.
What is a two-sample t-test?
Answer: A two-sample t-test is a statistical test used to compare the means of two independent samples to determine if they are significantly different from each other.
What is a paired t-test?
Answer: A paired t-test is a statistical test used to compare the means of two related samples, where each observation in one sample is paired with a corresponding observation in the other sample.
What is analysis of variance (ANOVA)?
Answer: Analysis of variance is a statistical technique used to compare the means of three or more groups to determine if there are any significant differences among them.
What is a chi-square test?
Answer: A chi-square test is a statistical test used to determine whether there is a significant association between two categorical variables.
What is regression analysis?
Answer: Regression analysis is a statistical technique used to model and analyze the relationship between a dependent variable and one or more independent variables.
What is correlation analysis?
Answer: Correlation analysis is a statistical technique used to measure the strength and direction of the linear relationship between two continuous variables.
What is the coefficient of determination (R-squared)?
Answer: The coefficient of determination, denoted as R-squared, measures the proportion of the variance in the dependent variable that can be explained by the independent variable(s) in a regression model.
What is the difference between a population parameter and a sample statistic?
Answer: A population parameter is a numerical value that describes a characteristic of a population, while a sample statistic is a numerical value that describes a characteristic of a sample.
Hypothesis Testing
Null Hypothesis and P-Value
What is the null hypothesis?
Answer: The null hypothesis is a statement that assumes there is no significant difference or relationship between variables in a population.
What is the alternative hypothesis?
Answer: The alternative hypothesis is a statement that contradicts the null hypothesis and suggests that there is a significant difference or relationship between variables in a population.
What is a Type I error?
Answer: A Type I error occurs when the null hypothesis is rejected, but it is actually true. It represents a false positive result.
What is a Type II error?
Answer: A Type II error occurs when the null hypothesis is accepted, but it is actually false. It represents a false negative result.
How are Type I and Type II errors related?
Answer: Type I and Type II errors are inversely related. Decreasing the probability of one type of error increases the probability of the other type.
What is the significance level in hypothesis testing?
Answer: The significance level, denoted as α, is the predetermined threshold used to determine whether to reject the null hypothesis. It represents the maximum probability of making a Type I error.
What is the p-value?
Answer: The p-value is the probability of obtaining a test statistic as extreme as the one observed, assuming the null hypothesis is true. It helps in deciding whether to reject or fail to reject the null hypothesis.
How is the p-value used in hypothesis testing?
Answer: If the p-value is less than the significance level (α), the null hypothesis is rejected in favor of the alternative hypothesis. If the p-value is greater than α, the null hypothesis is not rejected.
What does a p-value of 0.05 indicate?
Answer: A p-value of 0.05 (or less) indicates that there is a 5% (or less) chance of obtaining the observed result if the null hypothesis is true. It is a common threshold for determining statistical significance.
What does it mean if the p-value is greater than 0.05?
Answer: If the p-value is greater than 0.05, it suggests that there is not enough evidence to reject the null hypothesis. The results are not statistically significant.
What does it mean if the p-value is less than 0.05?
Answer: If the p-value is less than 0.05, it suggests that there is sufficient evidence to reject the null hypothesis. The results are considered statistically significant.
What is a one-tailed test?
Answer: A one-tailed test is a hypothesis test that checks for the difference or relationship in a specific direction, either greater than or less than. It has a directional alternative hypothesis.
What is a two-tailed test?
Answer: A two-tailed test is a hypothesis test that checks for the difference or relationship in either direction, greater than or less than. It has a non-directional alternative hypothesis.
How does the choice of one-tailed or two-tailed test affect the p-value?
Answer: The choice of one-tailed or two-tailed test affects the p-value calculation. In a one-tailed test, the p-value is halved because the test is focused on one direction. In a two-tailed test, the p-value remains as calculated.
How can you interpret a p-value?
Answer: The p-value provides a measure of the strength of evidence against the null hypothesis. A smaller p-value suggests stronger evidence against the null hypothesis, while a larger p-value suggests weaker evidence against the null hypothesis.
T-tests
What is the Student t-test?
Answer: The Student t-test is a statistical hypothesis test used to determine if there is a significant difference between the means of two groups or samples.
When should you use a Student t-test?
Answer: The Student t-test is typically used when the sample size is small, and the population standard deviation is unknown.
What are the assumptions of the Student t-test?
Answer: The assumptions of the Student t-test include independence of observations, normality of the data distribution, and homogeneity of variances between the groups.
What are the types of Student t-tests?
Answer: The two common types of Student t-tests are the independent samples t-test and the paired samples t-test.
What is the independent samples t-test?
Answer: The independent samples t-test compares the means of two independent groups or samples.
What is the paired samples t-test?
Answer: The paired samples t-test compares the means of two related groups or samples, such as before and after measurements on the same subjects.
What does the p-value in the t-test indicate?
Answer: The p-value in the t-test indicates the probability of obtaining the observed difference in means (or a more extreme difference) if the null hypothesis of no difference is true.
How do you interpret the p-value in a t-test?
Answer: If the p-value is less than the chosen significance level (e.g., 0.05), it suggests that there is evidence to reject the null hypothesis and conclude that there is a significant difference between the group means
What is the degrees of freedom in a t-test?
Answer: The degrees of freedom in a t-test represent the number of independent observations available for estimating the population parameters.
How does the sample size affect the t-test?
Answer: As the sample size increases, the t-test becomes more robust and less influenced by violations of normality or variance assumptions.
What is the critical value in a t-test?
Answer: The critical value in a t-test is a threshold value that separates the rejection region from the acceptance region based on the chosen significance level.
What is the effect size in a t-test?
Answer: The effect size in a t-test measures the magnitude of the difference between the group means and provides information about the practical significance of the results.
Can the Student t-test be used for non-parametric data?
Answer: No, the Student t-test assumes that the data follows a normal distribution. For non-parametric data, alternative tests like the Mann-Whitney U test or Wilcoxon signed-rank test should be used.
What is the difference between a one-tailed and a two-tailed t-test?
Answer: In a one-tailed t-test, the alternative hypothesis specifies a directional difference between the group means, while in a two-tailed t-test, the alternative hypothesis allows for a difference in either direction.
How do you calculate the t-statistic in a t-test?
Answer: The t-statistic is calculated as the difference between the sample means divided by the standard error of the difference. It measures how many standard errors the difference between the means is away from zero.
Chi-squared Tests
What is the chi-squared test?
Answer: The chi-squared test is a statistical test used to determine if there is a significant association between categorical variables.
When should you use a chi-squared test?
Answer: The chi-squared test is used when you have categorical data and want to test if there is a significant relationship or difference between the observed and expected frequencies.
What are the assumptions of the chi-squared test?
Answer: The assumptions of the chi-squared test include independent observations, a random sample, and an adequate sample size.
What is the null hypothesis in a chi-squared test?
Answer: The null hypothesis in a chi-squared test states that there is no association or difference between the categorical variables.
What is the test statistic in the chi-squared test?
Answer: The test statistic in the chi-squared test is calculated as the sum of squared differences between the observed and expected frequencies, divided by the expected frequencies.
How do you interpret the p-value in a chi-squared test?
Answer: If the p-value is less than the chosen significance level (e.g., 0.05), it suggests that there is evidence to reject the null hypothesis and conclude that there is a significant association between the categorical variables.
What is the degree of freedom in a chi-squared test?
Answer: The degree of freedom in a chi-squared test is calculated as (number of rows – 1) * (number of columns – 1).
Can the chi-squared test be used for continuous data?
Answer: No, the chi-squared test is specifically designed for categorical data. For continuous data, other tests such as the t-test or ANOVA are more appropriate.
What is the difference between the chi-squared test for independence and the chi-squared test for goodness of fit?
Answer: The chi-squared test for independence examines the relationship between two categorical variables, while the chi-squared test for goodness of fit compares observed frequencies to expected frequencies for a single categorical variable.
What is Yates' correction in the chi-squared test?
Answer: Yates’ correction is a small adjustment made to the chi-squared test statistic when analyzing 2×2 contingency tables. It helps to account for the approximation used in the chi-squared test.
Can the chi-squared test handle missing data?
Answer: No, the chi-squared test assumes complete data. If there are missing values, data imputation or other techniques must be used before performing the test.
What is the effect size measure in the chi-squared test?
Answer: There are several effect size measures for the chi-squared test, including Cramer’s V and Phi coefficient, which quantify the strength of the association between variables.
Can the chi-squared test be used for small sample sizes?
Answer: The chi-squared test can be used for small sample sizes as long as the expected frequencies in each cell are not too small (e.g., below 5). Otherwise, alternative tests like Fisher’s exact test should be considered.
What is the relationship between the chi-squared test and the chi-squared distribution?
Answer: The chi-squared test uses the chi-squared distribution as the reference distribution for calculating p-values and critical values.
How is the chi-squared test used in hypothesis testing?
Answer: The chi-squared test compares the observed frequencies in different categories to the expected frequencies, and based on the calculated test statistic and p-value, the null hypothesis is either accepted or rejected.
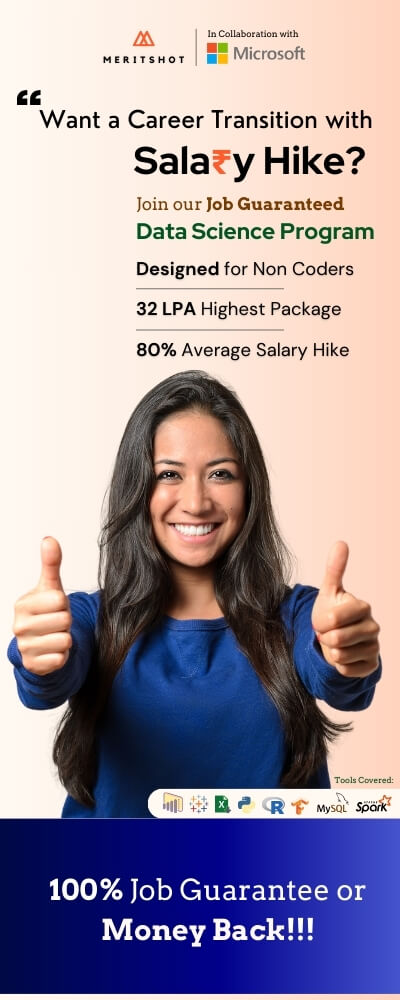